Created: 02/11/23
Last edited: 02/13/23
Hello Mycological Society Board,
I thank you for sending me some questions regarding the efficacy of DNA barcoding specimens with nanopore. I also thank you for allowing my response to your questions to be in the form of an open letter, rather than through private communications. This will allow information on this “new” technology to be disseminated in a more impactful and concise way, hopefully starting to allay the further dissemination of information that I believe to be based on an antiquated or misinformed understanding of the technology, protocols, and analytical pipelines.
Unfortunately, the information that was presented to your board is demonstrably false on every level. We have currently run over 10,000 specimens through the nanopore pipeline – the only large dataset of macrofungi that currently exists from the platform. Anyone interested in these topics could look at some actual results that have been documented and see that the information that was provided to your board is not just incorrect, but that there is no modicum of rational basis to support the error-rate claims that were outlined. Based on our publicly available data, the majority of the nanopore sequences match Sanger reference data with 100% similarity. It would be clear to anyone that works with the nanopore technology regularly that the individual(s) making these claims have not ever worked with the technology, much less for DNA barcoding, have not taken the time to understand the analytical pipelines that it employs, have not taken the time to review any nanopore barcoding results available, nor have they taken the time to review scientific literature on DNA barcoding with nanopore, and are making speculative claims with no underlying rational basis or evidence. I will outline current results we are seeing and relevant scientific literature later in this letter. As I understand the primary individual making these claims is a member of your board, I would encourage you to ask the individual to provide you with evidence to validate the information that was presented.
I apologize for the length and complexity of my response to the primary questions below, but I have tried to make it as basic and streamlined as possible. Hopefully it gives you some additional context, not only about your questions specifically, but also of the overall nanopore sequencing process in general.
“There was a lot of concern about the lack of accuracy of nanopore testing such as for the fungal samples we would be submitting. [Professional Mycologist] was saying there was about a 10% error rate with current nanopore testing. He/She felt that the error rate was so high that the results are unreliable. Can you help us understand if that error rate is about right and how the reliability of the method affects the reliability?”
To understand the error rate of nanopore, you must first understand how the outputs are presented, and a bit about the history of the technology. Sanger sequencing provides a single (or two) sequence file(s) that are examined to get a final result. With nanopore sequencing (as well as Illumina sequencing), there is not a single sequence from a given specimen as the primary output or one single error rate for the technology. There are hundreds, thousands, or tens of thousands of individual “reads” (sequences) that result for each specimen which goes through and analytical pipeline to produce a “consensus” sequence. Each of these resulting individual sequences has an average quality score that is assigned to it by the software based on how accurate it is believed to be. A quality score of a sequence is essentially equal to an estimated error rate for the sequence. A quality score of 10 would represent a 10% error rate and a quality score of 20 would represent a 1% error rate. Most resulting raw/unprocessed sequences from the nanopore protocol we utilize fall in this range. So for each specimen, there are thousands of resulting sequences with thousands of individual quality scores, often forming a quality score bell curve, which can visually represent the range of error rates for sequences for that specimen.
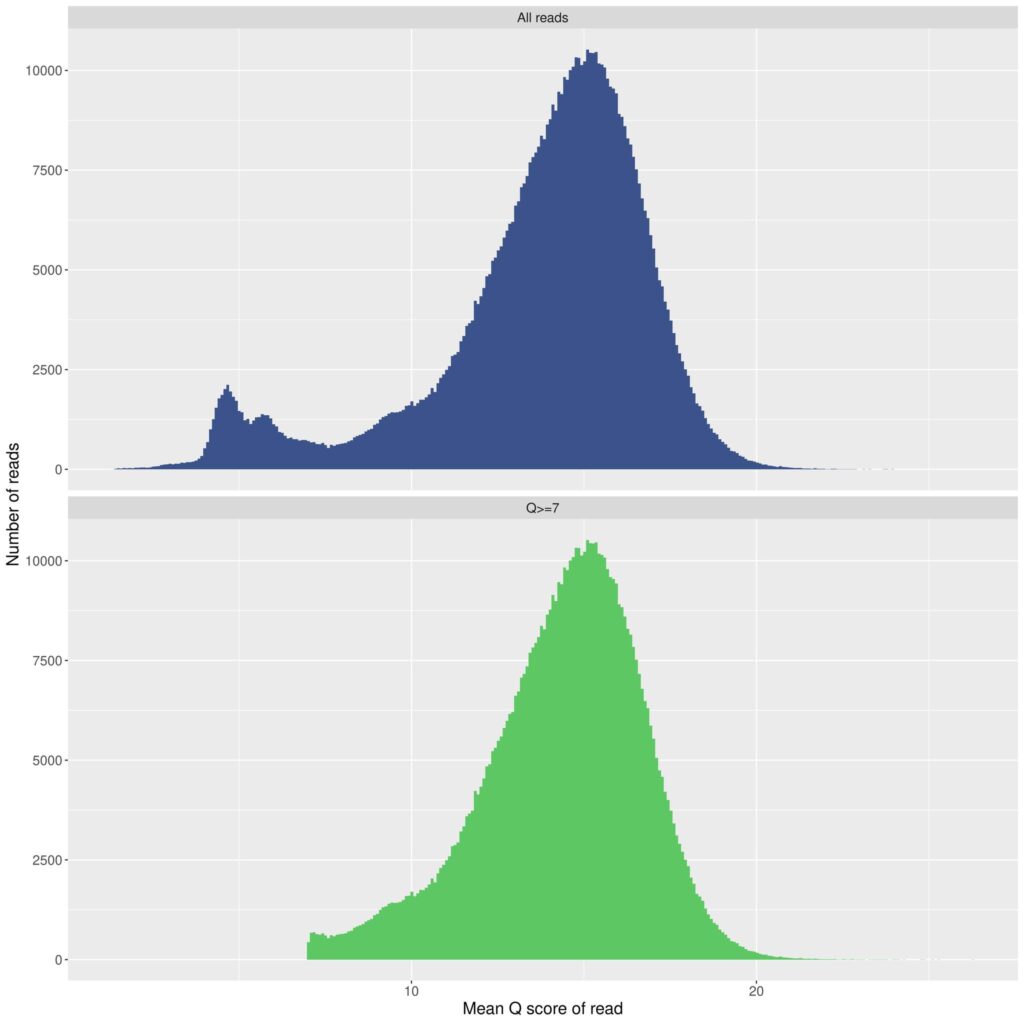
There are two main components (and several other minor components) that dictate the average error rate for a given nanopore run. The primary components are the version of the flowcell (the device that “reads” the individual bases) and the version of the chemistry that is utilized during preparation of the samples. Both the flowcell and chemistry are products that are produced by, and purchased from, Oxford Nanopore Technology (ONT). The most recent flowcell version, 9.4.1, was the primary product until late last year, at which time the updated 10.4.1 model was released. There were several upgraded aspects to the latest flowcell version, but one major improvement was the ability to capture “duplex” reads – examining the bases twice for each sequence – allowing the individual basecalls to be further validated. This is one component (among others) that has improved the accuracy in the recent flowcell design and that sparked my renewed interest in and adoption of this technology. The other main component that dictates the accuracy of the reads is the chemistry involved. To go along with the 10.4.1 flowcells, ONT released the v14 chemistry, which they market as “Q20+ Chemistry.” Any discussion of error rates for nanopore sequencing must be strictly aligned to the flowcell + chemistry combination that is being utilized for the project. The latest flowcell + chemistry combination gives significantly different results than previous combinations, as is true with any technology that iterates versions through time.
However, being unaware of recent improvements in technology could not justify the comments that were presented to your board, particularly that nanopore has a 10% error rate. The default setting on every nanopore pipeline I have seen, including from ONT itself, is to automatically discard all sequences that are of Q10 quality or under. This means that by definition, all sequences that are utilized must be better than a 10% error rate. Not only that, most sequences have to be significantly better than 10% error, as the Q10 filter is toward the left hand side of the bell curve of all of the reads that are present for a given specimen. Starting from a primary Q10 filter, it is always possible to have further filtering, depending on the needs of an individual project. As an example, it is possible to place your quality filter at Q20, which would mean that you are only using sequences with 1% or less error rate, and so it could be easily claimed that nanopore can produce an error rate of 1% or less. The average quality score for a run (the peak of the quality score bell curve) just depends on how the reads are filtered. The tradeoff here is that the higher you set the quality filter, the fewer the number of reads that make it through QC for use in your final analysis. So as with any sequencing technology, including Illumina, a judgement has to be made about the proper quality score filter for the needs of an individual project. This is typically based on what is being done with the resulting sequences, as well as the types of downstream analysis that are available to be employed for a particular goal. But overall, the average error rate of a nanopore run depends on how strictly you select the filter to be for the final reads; it is not a static percentage and can be altered depending on the needs of an individual project. If your final results are not looking reliable, you can continue to filter more stringently (discarding more reads with a low Q score), until you end up with the results that are needed for the goals of an individual project.
To understand this further, you will also need to understand a bit more about how the technology works to generate the final base calls. Nanopore works by sending DNA through small microscopic “pores” on the aforementioned flowcell. The primary output is a file of electrical signals from each individual pore on the flowcell. Software then interprets the changes in electrical signals saved in that file into individual base calls – A’s, G’s, C’s, and T’s – a step known as basecalling. ONT has a number of different options for calling the bases such as “high-accurate basecalling” and “super-accurate basecalling.” There are also many third-party basecallers such as “bonito” and “dorado.” Each of these basecalling algorithms has its own advantages and disadvantages, as well as their own error profile. This is another arena where there are algorithmic improvements occurring all of the time and improved basecalling models are being published regularly. Basecalling algorithms are significantly improving year-to-year, as new machine learning algorithms based on neural networks and other methods are implemented. This is a third area – along with flowcells and chemistry – that effect the error profile of the resulting reads and needs to be taken into account for any discussion of “nanopore error rates.”
Overall, there is nothing I am aware of that could justify the statement that a modern nanopore implementation has a 10% error rate, unless someone was referencing the average error rate for sequences that utilize an antiquated basecalling methodology, were not quality filtered, and that utilized antiquated flowcell models along with antiquated chemistry. The current default quality filtering alone ensures that every read in the results is better than Q10 (10% error rate). I would be happy to discuss additional details with anyone that would have further questions about the error rates.
But all of this is just step 1 – involving the technology/equipment and the basic preliminary processing; there are many more downstream aspects to nanopore sequencing that have an effect on the accuracy of the end product. I am including the following information for additional context, but it may be difficult to follow unless you are somewhat familiar with the specifics of next-generation sequencing. To understand these additional concepts, you must have a basic understanding of the “bioinformatic” processes that are utilized to create the end result. A summary follows:
The basic output to this point in the process would be hundreds of thousands of individual “reads” (sequences) in a single FASTQ file – a sequence file type that has individual sequences associated with quality scores (typically Q10-Q20) – representing 960 specimens in a pool, but with individual sequences not associated with any particular specimen. It is just a file with a pool of hundreds of thousands of sequences that are not associated with a specimen. The final steps turn this giant FASTQ file into individual sequences that are associated with individual specimens.
The first step in the final analytical pipeline is to utilize some software to “demultiplex” the reads. When PCR amplification is originally performed, the final sequences for each specimen have a unique 13 base pair long “tag” that is attached to each end of the sequence. When all of the PCR products are pooled (mixed) together for sequencing, typically 960 specimens at a time, these tags are also sequenced at the beginning and end of each read. The sequencing of these tags allow the final results to be reassociated with individual specimens through software filtering. This is called “demultiplexing.” Many programs are available for this – I use MiniBar. The results at the end of this step contain hundreds or thousands of sequences in individual FASTQ files, in a single labeled folder for each specimen being examined. From there, the sequences in the FASTQ files need to be merged together to form the best “consensus” sequence for the specimen.
Forming a consensus sequence can be relatively straightforward or complex, depending on the software programs that are used. I am currently using a program called NGSpeciesID for this process. Sequence pools in the FASTQ file are further filtered and subsampled. Primer and tag sequences are trimmed from the beginning and end of each sequence. A consensus sequence is then formed from all of the reads in the file associated with each specimen. The sequences in the file are aligned and the most frequent basecall across all of the reads is utilized; a significant improvement in accuracy can be attained by simply using the “consensus” base across all of the reads in the pool. As a secondary error-reduction measure, ONT has created a tool called medaka, that utilizes a neural network algorithm to further “polish” the consensus sequence utilizing the other reads in the pool. This final step also reduces the error profile of the final consensus sequence.
So even if a researcher is using the nanopore default parameters for quality, without any more stringent quality filtering, and the worst reads in a pool have 10% error (which only a small portion of the reads in a pool would have), there are still many other steps in the pipeline that ultimately reduce the error rate of the final nanopore consensus sequence to be equivalent to those produced by traditional Sanger sequencing.
I have an extensive collection of publicly-available internal data to backup the assessment that DNA barcoding with nanopore is equivalent to Sanger sequencing in terms of accuracy. My internal database has one of the largest collections of Sanger sequences available from eastern North America. When we are looking at nanopore sequences from this region, a vast majority of specimens that are sequenced have Sanger reference data that is available for comparison. To date, we have DNA barcoded over 10,000 specimens with nanopore. I would estimate that 90% of the individual specimens done in the last year with nanopore have previously generated Sanger reference data from other collections of the species that can serve to help validate the accuracy of the nanopore results. All one has to do is to look at the results/comparisons and the equivalency of Sanger is obvious prima fascia. The majority of nanopore sequences that are produced match previous Sanger reference data 100%. All of my data is publicly available online, free for anyone to look at to form their own judgements about the accuracy of nanopore sequencing, utilizing real-world data for macrofungi. A list of links with these data can be found at the end of this letter.
Finally, there is a significant amount of scientific literature to backup my assessment. I know of at least ten papers, across many different organismal groups, that conclude that DNA barcoding specimen-based amplicons results in sequences that are comparable to Sanger-generated sequences. The list of these papers can also be found at the end of this letter. I am aware of zero – 0 – papers that come to the opposite conclusion. If anyone is able to find any literature that backs up a claim that DNA barcoding specimen-based amplicons with nanopore are not Sanger-equivalent, please let me know, as I have yet to see ANY such finding. Also keep in mind, most of the papers outlined came to the Sanger-equivalent conclusion with now outdated 9.4.1 flowcells, V10 chemistry, and older basecalling models.
There are legitimate critiques regarding use cases for nanopore sequencing based on the error rate. In fact, concerns with the error rate was one of the primary reasons we delayed adoption of technology for so long. This would be a good juncture to briefly outline some differences in the efficacy of nanopore sequencing depending on the source of the DNA and the goals of the project – particularly sequencing whole genomic DNA vs. environmental DNA vs. vs. specimen-based amplified DNA (amplicons/barcodes) – as it will be important for much of our remaining conversation, and is contextually important. DNA amplification through PCR allows a researcher to turn a small amount of DNA that is present in a sample into a very large number of copies of targeted areas (such as the ITS region) that have been shown to be useful for identifying specimens. When you have a large number of copies of a very small region of DNA, that allows individual random errors in a sequence (the most common type with nanopore) to stand out when comparing it to all of the other resulting sequences in the pool. If you only have a small number of sequences in your pool, such as when looking at genomic DNA or environmental DNA, there are far fewer options for validating the correct base calls in an individual read against the other corresponding reads. Stated another way, when you are dealing with amplicons, the accuracy of any one individual read becomes less important, because you have many more sequences of the same targeted region to validate the proper basecall. When you are dealing with genomic DNA with a lower sequencing depth/read count for each individual region of the genome, the accuracy of any one individual read becomes far more important, as you have far fewer points of comparison to validate the accuracy of an individual basecall. When you are primarily looking at amplified DNA of a known specimen, with thousands of copies of the same small segment, there are not only many more options to validate the resulting sequence against other sequences in the pool, but also to validate through external sequences of the species that have previously been produced (external reference data). The bioinformatic software programs that are available to fully analyze each type of sample is also very different depending on what source the original material is from. Quite often people quote raw nanopore error rates from researchers who are examining genomic DNA rather than amplified DNA that has gone through an extensive error-correcting/filtering pipeline. This is comparing apples to oranges, as there are many more analytical options for reducing the error rate and validating the sequences for amplicons than there are for validating the final sequences coming from genomic DNA. At this point in time, I would not be an advocate for doing genomic work or examining environmental DNA with nanopore, because of the error rate and the low read count/number of validation sequences that are available for those types of goals, especially once the requisite quality score filters are applied.
I would finally note here, that while nanopore sequencing has not been used for extensively for fungal analysis to this point, it has been extensively used for other research domains, particularly for COVID analysis. Many of the protocols and bioinformatic analytical pipelines are the same or similar to other methods that have been extensively documented within the scientific literature of virology.
“A major concern was the lack of a chromatogram, such as with Sanger, so the results couldn’t be looked at to see what you’re dealing with and clean things up”
While it is true that there is no chromatogram with nanopore, the process does generate its own version of raw data files. There is actually more information available from the raw data files of nanopore than a Sanger chromatogram has; with even more opportunities than Sanger to “see what you are dealing with and clean things up.”
One preliminary note, the vast majority of mycologists do not make their Sanger chromatograms publicly available. This is an inherent issue with the reference data available in most public repositories, most notably, GenBank. There is no way to assess any sequence that is contained within GenBank for quality. Nor if contacted, do most mycologists have all of their past chromatograms in a readily accessible internal structure that can be accessed, internally or externally, nor are many willing to readily share them. So for vast majority of sequences that are already in GenBank, there is NO ability “to see what you’re dealing with and clean things up.”
The one major exception is every Sanger sequence that I have ever personally produced, which likely equals 5-10% of all Sanger sequences that have ever been generated globally for macrofungal specimens. Each of my Sanger sequences has a publicly accessible forward and reverse AB1 file. This allows future researchers to have the raw data instantly for each Sanger sequence record. I know of no other researcher within mycology who has found this to be an important aspect of their research. I have always found this to be important, as editing a Sanger-based DNA sequence from AB1 trace files can be highly subjective and prone to error. Regarding the public accessibility of raw DNA data, I hold the same philosophy for nanopore sequencing. Each nanopore sequence that is produced has a FASTQ file associated with it that is publicly accessible. This file contains all of the component sequences that form the final consensus sequence, as well as the quality scores for each of these component reads. Since the raw nanopore files (that contain the electrical signals) will also be able to be improved long into the future and may be valuable to future researchers, these will ultimately be deposited in the NCBI’s Sequence Read Archive. This way if a new algorithm is produced 50 years from now that is exponentially better than what we have now (which is a likely scenario), all of the raw data will be able to be reanalyzed and new results produced. While nanopore data is already comparable to Sanger in terms of accuracy, it also has the ability to be updated in perpetuity as new methodologies become available. With Sanger, most of the raw data is not even publicly available, much less able to be reassessed as the needs of an individual researcher should often require.
Even though nanopore does not produce chromatograms, it produces files of electrical signals for basecalling and FASTQ files that show the quality of each individual basecall that forms the consensus, which in combination could be considered more valuable for determining the accuracy of any nanopore generated sequence than a chromatogram is for Sanger. Interpreting nanopore sequences and improving analytical pipelines is a significant area of ongoing research, that will continue to improve in perpetuity.
“We were told that the current efforts with nanopore sequencing en masse is problematic because it is viewed as flooding GenBank with noise that does more harm than good because it is obscuring the signals”
It is very surprising that any professional mycologist would make this claim. First, if you look at what is already in GenBank, it is full of “environmental sequences” – often produced by Illumina sequencing – that are not associated with any specimen, do not have quality scores reported, and do not have the raw data available to validate. They are very frequently riddled with errors. There are tens of thousands of them in GenBank. I suppose one could say these environmental sequences do “more harm than good because it is obscuring the signals,” (which I would still disagree with), but there is a simple solution. If you do not want them included, you simply filter them from your results. The same could be said of nanopore sequencing. Even if you believed what the statement purports, that they are just noise, the solution is simple – just filter them out. Every GenBank record has the sequencing technology that was employed listed within the accession. The advantages of the nanopore sequences we produce is that all of the raw data to form the consensus sequence is publicly available and every sequence has a publicly available quality score for each individual base that is being reported. I would actually echo the inverse of this statement, and claim that the majority of the remainder of GenBank is more problematic, as without the raw data files, the individual bases being reported by researchers worldwide are not able to be validated when differences are spotted among the available reference data. This would appear to be a larger issue for “obscuring the signal” as editing errors or erroneous sequences are not able to be clearly spotted or flagged for research purposes.
But our overall goal is to clearly “flood” GenBank with significantly more amounts of reference data than it currently has.
Members of your mycological society are still very welcome to participate in the 2023 Continental MycoBlitz, even if your board decides not to fund any additional sequencing. We will be holding these events every year until a reasonably comprehensive mycoflora of North America is obtained. This will give some time for your board to digest the information that has been provided and to review local results that are generated by those who decide to participate, before committing to playing any larger role. I thank you for your time and consideration, and hope this experience does not sour the initial enthusiasm for the project that some members of your board previously held.
Best,
Steve Russell
Papers supporting an equivalent error rate for nanopore amplicon-based sequencing vs. Sanger sequencing:
“We document that MinION barcodes are virtually identical to Sanger and Illumina barcodes for the same specimens (> 99.99%) and provide evidence that MinION flow cells and reads have improved rapidly since 2018.” https://bmcbiol.biomedcentral.com/articles/10.1186/s12915-021-01141-x
“Using the R9.4.1 flow cell, IGS sequence identities averaged 99.57% compared to Sanger sequences of the same region. When the newer R10.3 flow cell was used, accuracy increased to 99.83% identity compared to the same Sanger sequences. Nanopore sequencing errors were predominantly in regions of homopolymers, with G homopolymers displaying the largest number of errors and C homopolymers displaying the least. Phylogenetic analysis of the nanopore- and Sanger-derived sequences resulted in indistinguishable trees.” (fungi) https://journals.asm.org/doi/full/10.1128/JCM.01972-20
“perfect concordance of the nanopore sequence mutations to Illumina and Sanger data” (fungi) https://sfamjournals.onlinelibrary.wiley.com/…/lam.13516
“Our methods successfully identified yeasts at the species level rapidly (approximately 3.5 hours from DNA extraction to data analysis), and with high accuracy that is comparable to Sanger sequencing (average percentage identity >98.9%)” (Fungi) https://onlinelibrary.wiley.com/doi/abs/10.1002/jib.639
“In this study, we have successfully produced 96×7 MLST alleles using MinION and validated 359 alleles entirely consistent with Sanger sequencing. ” https://www.ncbi.nlm.nih.gov/pmc/articles/PMC7200061/
“PhyML phylogeny (100 bootstraps) of NP2 samples with both Sanger (shown in blue) and Oxford Nanopore (shown in brown) sequences of the same individuals, showing perfect congruence between the two datasets.” https://link.springer.com/article/10.1186/s13071-022-05255-1
“Comparison of both sequencing workflows showed a near perfect agreement with no false negative calls” https://journals.plos.org/plosone/article?id=10.1371/journal.pone.0265622
“The maximum observed sequence divergence (MinION — Sanger) across 50 replicates and six species was 0.24%, or 1 base for the 420 bp mtDNA cyt b sequence;” https://portlandpress.com/…/465/920825/etls-2020-0287c.pdf
“Partial RdRP sequences were successfully amplified and sequenced from 82.46% (47/57) of specimens, ranging from 75 to 100% by virus type, with consensus accuracy of 100% compared with Sanger sequences available (n = 40).” https://virologyj.biomedcentral.com/…/s12985-020-01454-3
“In summary, our nanopore sequencing-based platform for HIV-1 genome analysis enables us to determine viral RNA genome sequences in patient plasma and to efficiently distinguish RFs from mixed genotypes in dual-infection samples. Unlike conventional sequence determination by Sanger sequencing, which is a prerequisite for gene fragment cloning or limited dilution, this nanopore sequencing platform is simple and efficient for determining the near-full-length HIV-1 genome.” https://journals.asm.org/doi/full/10.1128/spectrum.01507-22
Project links that contain specimen-based DNA barcodes:
https://mycomap.com/projects/ont-indiana-first-300
https://mycomap.com/projects/ont002-second-run-nanopore
https://mycomap.com/projects/ont003-third-run-nanopore
https://mycomap.com/projects/ont004-fourth-run-nanopore
https://mycomap.com/projects/ont005-fifth-run-nanopore
https://mycomap.com/projects/ont006-sixth-run-nanopore
https://mycomap.com/projects/ont007-seventh-run-nanopore
https://mycomap.com/projects/ont008-eighth-run-nanopore
https://mycomap.com/projects/ont009-ninth-run-nanopore
https://mycomap.com/projects/sigrid-jacob-ont009
https://mycomap.com/projects/sequencing-course-ont010
https://mycomap.com/projects/sigrid-jakob-ont010
https://mycomap.com/projects/jacob-kalichman-ont010
https://mycomap.com/projects/danny-miller-dec-2022
https://mycomap.com/projects/sequencing-course-ont011
https://mycomap.com/projects/danny-miller-ont011
https://mycomap.com/projects/alfredo-justo-ont011
https://mycomap.com/projects/ken-sanderson-ont011
https://mycomap.com/projects/nama-2022-potosi-mo-ont008
Response from [Professional Mycologist]
Personally identifiable information has been redacted by SDR.
Submitted 2/13/23
Greetings [Mycological Society] Board Members (and [amateur mycologist], with Mr. Russell CCd):
My apologies to Mr. Russell for not answering his email request to privately discuss Nanopore issues more promptly – I was out this past weekend, so I will present my comments here openly and directly.
I will try to avoid “complexity” and try to keep my comments brief and to the point.
In my discussion with the [Mycological Society] Board, I opened by mentioning the importance of reproducibility as a core tenet to science.
While I highlighted the fact that I am very pleased with and do encourage the mobilization of ‘citizen science’ in these types of efforts, one of my mentioned concerns was for the long term preservation of specimens generated in such efforts, especially in a time when Fungaria have limited resources.
In my comments to the Board, I lauded the efforts of [amateur mycologist], who is systematically documenting the [project name], one specimen at time, and depositing these high quality specimens in [professional herbarium] along with their related generated sequences and matching chromatograms for marker genes of interest (e.g., the fungal barcode ITS). In this way, we are gaining important knowledge of [state] fungi, the specimens are being collected in a targeted manner (i.e., filling in knowledge gaps where key voucher specimens are lacking and not overrunning [herbarium] with pointless, replicated specimens that take up valuable financial resources), and this is all done in a manner that is reproducible (the specimens are publically available via [herbarium] for the long run) and that holds up to scrutiny (i.e., all individual specimen sequences are match one-to-one to chromatograms that document the sequence quality).
I would be interested in knowing about the specific long term preservation plans for specimens generated in these efforts, their public availability, and how individual high quality specimens are linked directly to their matching individual generated sequences for the long term.
In my comments to the board, I compared Nanopore to the industry standard for high-throughput sequencing, which is Illumina, and I discussed quality (Q) scores for that technology vs. nanopore (discussion of Illumina Q scores can be found here: https://www.illumina.com/content/dam/illumina-marketing/documents/products/technotes/technote_understanding_quality_scores.pdf).
I cited a paper that suggests that actual error rates may “approach” 10% (6-8% as I mentioned):
https://journals.plos.org/plosone/article?id=10.1371/journal.pone.0257521
I mentioned that Illumina quality targets are typically in the Q30-Q40 (i.e., 1 in 1000 to 1 in every 10,000 base-pairs of DNA) range, which I personally believe to be only potentially acceptable for taxonomic work, as they are still “blackboxish” with individual specimens ultimately still not linked one-to-one to verifiable sequences, but rather to a pool of mixed sequences.
While current consensus sequence quality control methods certainly can go a long way in improving read quality, as I mentioned, the underlying Nanopore technology is still orders of magnitude “inferior” to Illumina sequencing (I believe the cited “Figure 1” from “our thirteenth nanopore run” shows quality scores largely fall within the Q10-Q20 range, i.e., 1 in 10 to 1 in 100, with the highest frequency at ca. Q15, i.e., noticeably worse than 1 in 100).
Given my age (I’m not getting any younger), I admit that I am a bit “Old School”, in that I would still like to see long term preservation in public fungaria of high value specimens (types or targeted vouchers) that are individually linked to high quality, verifiable sequences (but who am I to say as I am no longer really that “active” in field mycology).
This is just my personal opinion of course, but I would also really like to see that, at very best, 1 in 100 overall error rate associated with these Nanopore quality scores move up, especially given the nature of high-throughput marker gene sequencing and the typical bp length of fungal ITS.
In my discussion with the [mycological society] Board, I mentioned the problem in GenBank with third-party annotations [see: Bidartondo et al. 2008. https://doi.org/10.1126/science.319.5870.1616a] and I relatedly mentioned the utility of UNITE (Nilsson et al. 2019: https://doi.org/10.1093/nar/gky1022) and PlutoF (Abarenkov et al. 2010: https://doi.org/10.4137/EBO.S6271) as a neat way to validate “true” sequence identities with their associated names [and see Kõljalg et al. (including yours truly) 2013: https://doi.org/10.1111/mec.12481 for more information on sequence-based identification of fungi].
Considering Mr. Russell’s confidence, I would also encourage him to publish the method validation results of the “10,000 specimens through [his] the nanopore pipeline” in the ultimate scientific (now largely open) public forum, which is the peer-reviewed journal process.
Feel free to post my comments here to the “draft – open letter” (I’ve kept a copy for my personal records) on Mr. Russell’s personal website for the final version of the “open letter”.
SDR Response
Personally identifiable information has been redacted by SDR.
Submitted 2/13/23
Hello all,
And thank you [professional mycologist] for the response. I have been recently intending to summarize the nanopore error discussion in some way, as it is a recurrent issue that pops into discussions periodically, often with substantive misinformation and/or comments that often lack the required context for people to understand it in a meaningful way. The questions from the [mycological society] board presented a great opportunity to put many of my thoughts in one place. Even if we would have talked, the response to the [mycological society] board questions would still likely have been in the form of an open letter. I would prefer to not publicly name the [mycological society] mycological society or any other individuals in my letter. Publishing these additional comments would do that, but I can append them if you want me to, reacted or unredacted. I will remove the draft and lock it in.
As for the original open letter itself, I do not believe I would alter any of my original comments based on this reply. But in turn, I do think I agree with the bulk of [professional mycologist’s] comments here.
Reproducibility is clearly core to science. Retaining specimens is also a core concept of my philosophy, and we have tens of thousands of specimens in the Hoosier Mushroom Society’s herbarium. This is where the bulk of the specimens from the project would be stored for the foreseeable future. Most institutional herbaria lack the staff, space, and resources to process thousands of specimens. Our goal is 100k new specimens over the next ten years. So there are two options. One is to take the steps mentioned of a traditional, slow, methodical, targeted collection manner that herbaria can handle, knowing that this “filling in the gap” approach will miss an incalculable amount of interesting diversity. As we now know from Indiana, most of what we thought we knew, we were actually wrong about or had an incomplete picture of. This would also mean abandoning any attempt at actually documenting the full diversity of North American macrofungi (or even get a handle on regional diversity; we now estimate it will take 40,000 collections to get a reasonably comprehensive survey of Indiana macrofungi).
Another course would be to alter traditional models of collecting and storing specimens. We have done this on the collecting side with the online foray model. Our current model on the storage side is to maintain specimens locally in our mycological society’s herbarium. As researchers request specimens, there is unfettered and fast access to send them splits as needed. All of the specimens are available to be resampled locally for DNA as needed in very short time frames. And amateur mycologists have access to all of the specimens, something that is often restricted in professional institutions. Specimens will ultimately make their way into professional herbaria as they are utilized in the scientific literature in some way. This model also keeps replicated and uninteresting specimens out of the primary workflow for herbaria. Eventually, all specimens from the HMS will end up in a professional herbarium. But only one that will agree to an “open access” policy – where specimen requests will be honored for amateurs and professionals alike. We already have several herbaria considering accepting specimens under this precondition. I would anticipate that the bulk of our collections will never be formally accessioned into an herbarium, although they would be physically located at one. Something akin to the specimens from the Northwest IN Monitoring initiative, where thousands of specimens are physically at the Field Museum, where they are indexed and accessible, but not formally accessioned into the collection. They would be stored in a compact, space saving format, utilizing our current numbering system, and people would find out their location through the iNaturalist record or the GenBank accession. I suspect most of the specimens would never need to be looked at again, as they have a public sequence, but the specimen would still exist if the need arose.
For specimen linkage to sequences, images, and collection info, iNaturalist is our central hub for this. It is also where voucher number and herbarium location information is stored. All our GenBank accessions are linked to an iNat or MO record. As I mentioned in the open letter, all nanopore sequences that are produced are linked to publicly available raw data (FASTQ files). Are all the chromatograms for [professional herbarium] specimens publicly available? If not, that means they cannot be subjected to any scrutiny, unless they are resequenced.
As every project has different goal orientations, different models for preserving specimens should be expected, depending on the goals and the needs of an individual project. I believe the model we are working towards is the only option to actually produce a comprehensive survey of macrofungi in North America.
As for error rates, I would agree completely that nanopore is inferior to Illumina. It probably won’t be comparable for another 5 years or so. But that’s not really the question at the core of this discussion. As we are talking about barcoding, the question is whether hundreds or thousands of Q15 nanopore reads of a given specimen can be processed using bioinformatics and error reduction algorithms to produce a Sanger-quality end result. Quoting a 6-8% error rate is not really useful context to provide for the discussion. That is the error rate for single reads, from previous generations of flowcells and chemistry, without being processed through error-correcting algorithms or forming a consensus based on hundreds or thousands of other reads based on the same specimen. I submit that the current data we have generated, as well as the body of scientific literature generated to date clearly suggests that DNA barcodes from nanopore can be Sanger-equivalent. Further, as the bioinformatics continues to progress, the most frequent types of errors that are seen (conflated hapotypes, short repeats, homopolymer repeats, etc) will able to be further parsed out more accurately in the future. Even from previous data sets. But I do not think there will be a need to revisit old datasets with any regularity, as the majority of nanopore consensus sequences match a previously generated Sanger sequence at 100% similarity. [redacted due to a current area of ongoing research]
UNITE and PlutoF surely have a place, but they also have significant limitations that I won’t go into here.
I have no current plans to publish our results thus far in peer-reviewed journals. It takes a lot of time for little benefit, when there is so much work left to do. I have published a full protocol for barcoding fungal specimens with nanopore (It still needs to be updated for the latest chemistry and flowcells). I have also published an article in the recent MSA newsletter on nanopore sequencing, introducing the professional community to the results I am seeing. While I personally have little intent to publish extensively, I have generated DNA barcode data for many professional and amatuer mycologists that do have an inclination to publish, so I am sure you will be seeing many papers on these topics in subsequent years. I will probably take the time to publish once we get to the 100,000 specimen milestone, and we are able to make an assessment about how much more work we will need to do to get to a reasonably comprehensive mycoflora of North America.
Overall our goal with the Continental MycoBlitz 2023, which will continue every year in perpetuity, is to have high quality sequences linked to individual specimens. I believe that is a goal we all share. It would be great to have the [mycological society] club help out this year, as I find your region to harbor some of the most interesting diversity in the country (and often overlapping with Indiana), but I understand if you would like to adopt a wait-and-see approach. I am sure there will be many people from [state] that decide to participate, and we will process their specimens regardless of the outcome of this conversation.
Thank you all for your time,
Steve Russell